Mastering Time Series Graphs: A Comprehensive Guide
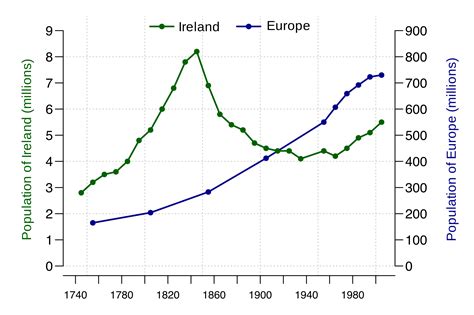
Time series graphs are powerful tools used to visualize and analyze data points that are collected over regular intervals of time. These graphs provide a visual representation of trends, patterns, and variations in data, making them invaluable for businesses, researchers, and analysts alike. In this comprehensive guide, we will delve into the intricacies of time series graphs, exploring their various types, applications, and the insights they can offer. By understanding the nuances of these graphs, you'll be equipped to make informed decisions and uncover valuable patterns within your data.
Unraveling the Complexity of Time Series Graphs
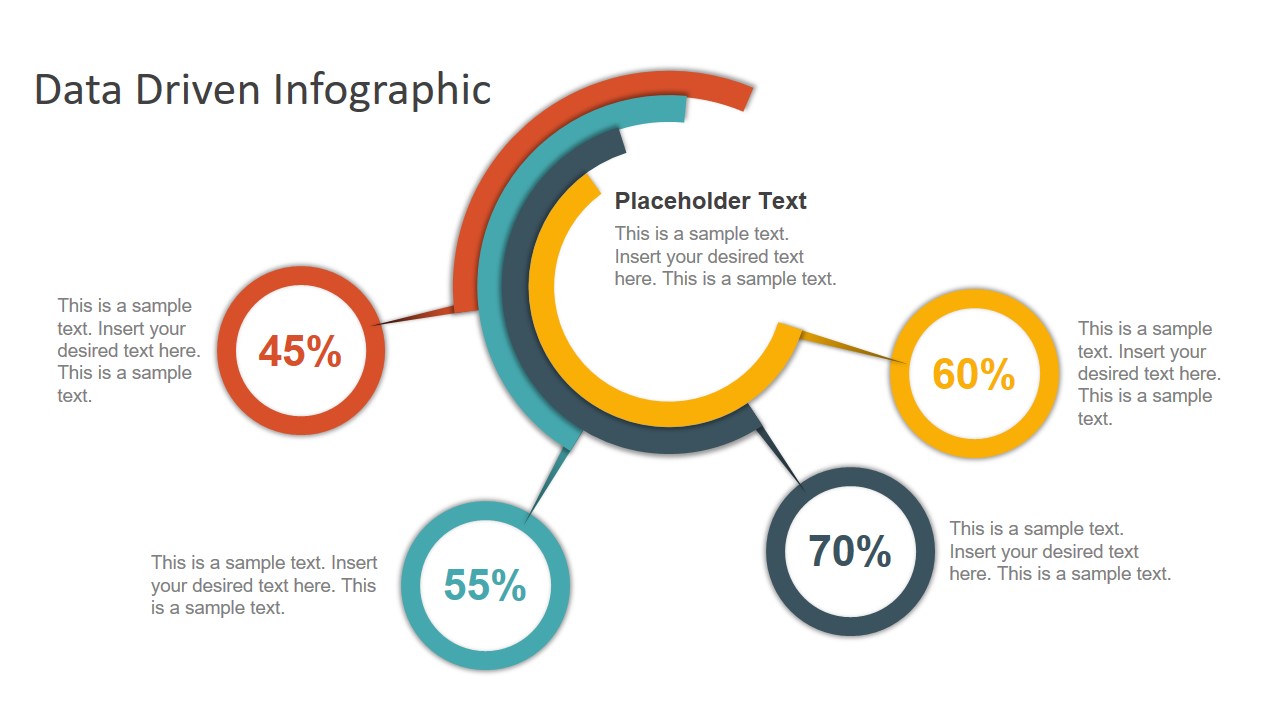
Time series graphs are more than just a collection of data points plotted over time. They are a dynamic and insightful tool that can reveal hidden trends, cycles, and anomalies within your data. Whether you’re tracking stock market trends, monitoring climate patterns, or analyzing sales data, time series graphs offer a unique perspective that can shape your understanding and inform your strategies.
Understanding the Basics: Types of Time Series Graphs
The first step in mastering time series graphs is to grasp the different types and their unique applications. Here’s an overview of the most common types of time series graphs and their specific use cases:
- Line Graphs: Line graphs are the most fundamental type of time series graph. They connect individual data points with lines, making it easy to visualize trends and identify patterns over time. Line graphs are ideal for showcasing continuous data, such as temperature changes or stock prices.
- Bar Graphs: Bar graphs represent data as vertical or horizontal bars, with the length of the bar corresponding to the value of the data point. This type of graph is excellent for comparing different categories or groups of data over time. For instance, you can use bar graphs to compare sales figures across different months or years.
- Area Graphs: Area graphs are similar to line graphs, but they fill the area beneath the line with color or shading. This visualization technique is useful for emphasizing the cumulative effect of data over time. Area graphs are often used to highlight the growth or decline of a particular metric, such as the total revenue over a period.
- Scatter Plots: Scatter plots are used when you have two sets of data points that you want to compare. Each data point is plotted as a dot on the graph, and the distribution of these dots can reveal interesting relationships and correlations. Scatter plots are particularly useful for identifying trends or patterns in complex datasets.
- Histogram: Histograms are a type of bar graph that represents the distribution of data within specific ranges or intervals. They are useful for understanding the frequency or density of data points within a given time frame. Histograms can help identify patterns or anomalies in data distribution.
Each type of time series graph has its own strengths and is suited to different types of data and analysis. Understanding which graph type to use for your specific data and objectives is a key aspect of mastering time series analysis.
Analyzing Time Series Data: Techniques and Insights
Once you’ve chosen the appropriate graph type for your data, the next step is to delve into the analysis. Here are some key techniques and insights you can gain from time series graphs:
- Trend Analysis: Time series graphs allow you to identify long-term trends in your data. By examining the overall direction and slope of the graph, you can determine whether your data is generally increasing, decreasing, or remaining stable over time. This can help you make predictions and plan accordingly.
- Seasonal Patterns: Many datasets exhibit seasonal patterns, where certain trends or variations occur regularly at specific times of the year. Time series graphs can help you identify these patterns, which can be crucial for businesses or organizations that plan their strategies based on seasonal fluctuations.
- Cyclical Patterns: Beyond seasonal patterns, some datasets exhibit longer-term cyclical patterns. These patterns may repeat over multiple years or decades. By analyzing time series graphs, you can identify and understand these cycles, which can provide valuable insights for long-term planning and forecasting.
- Anomalies and Outliers: Time series graphs can also help you detect anomalies or outliers in your data. These are data points that significantly deviate from the overall trend or pattern. Identifying anomalies can be critical for understanding unexpected events or issues that may impact your operations or strategies.
- Forecasting: One of the most powerful applications of time series graphs is forecasting. By understanding past trends and patterns, you can make informed predictions about future data points. This is particularly valuable for businesses that need to plan for future demand, sales, or resource allocation.
The insights gained from time series analysis can have a significant impact on decision-making and strategic planning. By recognizing and interpreting the patterns and trends in your data, you can make more accurate predictions, optimize your operations, and gain a competitive edge.
Advanced Techniques: Transforming Your Data into Actionable Insights
While basic time series analysis is already powerful, there are advanced techniques that can further enhance your understanding and insights. These techniques include:
- Decomposition: Decomposition is a technique used to break down a time series into its constituent components, such as trend, seasonality, and residuals. By isolating these components, you can gain a deeper understanding of the underlying factors influencing your data.
- Smoothing: Smoothing techniques, such as moving averages or exponential smoothing, can help reduce the impact of random fluctuations in your data. This can make it easier to identify underlying trends and patterns, especially in datasets with high variability.
- Time Series Forecasting Models: There are various statistical and machine learning models specifically designed for time series forecasting. These models, such as ARIMA (AutoRegressive Integrated Moving Average) or Prophet, can provide more accurate predictions by accounting for complex patterns and trends in your data.
- Data Aggregation and Resampling: Depending on your data and analysis objectives, you may need to aggregate or resample your data to obtain a more meaningful and manageable time series. This process involves combining multiple data points into a single aggregated value or resampling the data at a different frequency.
Advanced techniques like these can significantly enhance your ability to extract meaningful insights from time series data. They allow you to dive deeper into your data, uncover hidden patterns, and make more precise predictions and decisions.
Case Studies: Real-World Applications of Time Series Graphs

To illustrate the power and versatility of time series graphs, let’s explore some real-world case studies where these graphs have been instrumental in driving insights and decision-making.
Case Study 1: Stock Market Analysis
In the world of finance, time series graphs are essential tools for analyzing stock market trends and making investment decisions. For instance, a financial analyst might use line graphs to track the historical price movements of a particular stock over the past year. By examining the trend and identifying key turning points, they can make informed predictions about the stock’s future performance.
Additionally, the analyst might use bar graphs to compare the performance of different stocks or indices over time. This can help them identify sectors or industries that are performing well or struggling, which can influence their investment strategies.
Case Study 2: Climate Monitoring
Time series graphs are also vital in the field of climatology, where scientists and researchers track and analyze climate data to understand long-term trends and patterns. For example, they might use line graphs to plot the average global temperature over the past century. This graph would reveal the overall warming trend, helping scientists make predictions about future climate conditions and the potential impacts on ecosystems and human societies.
Area graphs can also be used to illustrate the cumulative effect of climate variables, such as the total rainfall or snowfall over a period of time. This visualization can help researchers understand the seasonal distribution of precipitation and its potential impacts on water resources and agriculture.
Case Study 3: Sales and Marketing Analytics
Businesses heavily rely on time series graphs to analyze sales and marketing data. For instance, a marketing team might use bar graphs to compare sales figures across different product categories or regions over time. This analysis can help them identify which products or regions are performing well and which ones require additional attention or strategic adjustments.
Scatter plots can be particularly useful in sales analytics to identify correlations between different marketing campaigns and their impact on sales. By plotting campaign spend against sales figures, the team can assess the effectiveness of their marketing strategies and make data-driven decisions for future campaigns.
The Future of Time Series Graphs: Advancements and Innovations
As technology and data analytics continue to evolve, the field of time series analysis is also experiencing significant advancements and innovations. Here’s a glimpse into the future of time series graphs and the potential directions they may take:
- Big Data and Time Series Analysis: With the proliferation of big data, time series analysis is becoming increasingly complex and powerful. Advanced algorithms and machine learning techniques are being developed to handle large-scale, high-frequency time series data, allowing for more accurate predictions and insights.
- Real-Time Analytics: The ability to analyze and visualize time series data in real-time is a game-changer for many industries. Real-time analytics can provide immediate insights and notifications when anomalies or critical events occur, enabling faster decision-making and response times.
- Interactive Visualizations: Static time series graphs are powerful, but interactive visualizations take this concept to the next level. Interactive graphs allow users to explore and manipulate data, drill down into specific time frames, and customize their visualizations to gain deeper insights. This interactivity enhances user engagement and understanding of complex data.
- Integration with AI and Machine Learning: The integration of time series analysis with artificial intelligence (AI) and machine learning (ML) algorithms is opening up new possibilities. These technologies can identify patterns and trends that might be too complex or subtle for human analysts to detect, leading to even more accurate predictions and insights.
- Cloud-Based Time Series Databases: Cloud computing is transforming the way time series data is stored and analyzed. Cloud-based time series databases offer scalable, cost-effective solutions for managing and analyzing large volumes of time series data, making advanced analytics accessible to a wider range of organizations.
The future of time series graphs is exciting, with advancements in technology and data analytics paving the way for even more powerful and insightful visualizations. As we continue to push the boundaries of what's possible, time series analysis will play an increasingly vital role in driving decision-making and shaping the future of numerous industries.
How do I choose the right type of time series graph for my data?
+
The choice of graph type depends on the nature of your data and your specific analysis goals. Line graphs are great for continuous data, bar graphs for comparisons, area graphs for cumulative effects, and scatter plots for relationships. Consider the story you want to tell with your data and choose the graph that best highlights those insights.
Can time series graphs help predict future trends or patterns?
+
Yes, time series graphs are a powerful tool for forecasting. By analyzing past trends, patterns, and cycles, you can make informed predictions about future data points. Advanced forecasting models and techniques can further enhance the accuracy of these predictions.
What are some common challenges in working with time series data?
+
Challenges include dealing with missing data, outliers, and seasonality. Missing data can be addressed through imputation techniques, while outliers may require careful consideration and potential removal or transformation. Seasonality can be modeled and accounted for in forecasting models.