skip.

Welcome to a comprehensive exploration of the world of machine learning, a field that has revolutionized the way we interact with technology and has immense potential to shape the future. In this in-depth article, we will delve into the intricacies of machine learning, its applications, and its impact on various industries. From its humble beginnings to its current state of rapid evolution, we will uncover the secrets and insights that make machine learning an essential tool in the digital age.
The Evolution of Machine Learning: A Historical Perspective
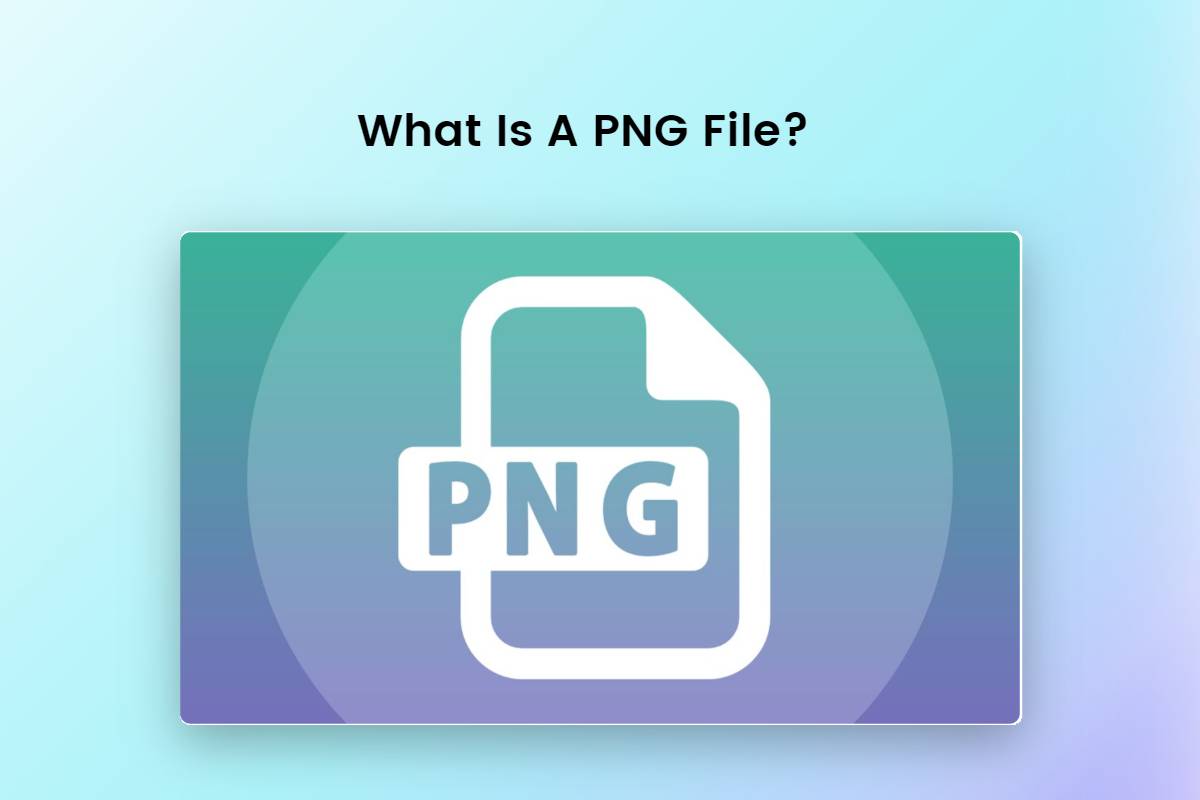
Machine learning, often referred to as ML, is a branch of artificial intelligence (AI) that focuses on the development of algorithms and models that enable computers to learn and make predictions or decisions without being explicitly programmed. It has come a long way since its early concepts and theories, which can be traced back to the 1950s.
The roots of machine learning lie in the efforts of pioneering researchers like Alan Turing and Arthur Samuel, who laid the foundation for the concept of "learning machines." Turing's work on computing machinery and intelligence, published in 1950, introduced the idea of machines that could learn and reason, sparking the imagination of computer scientists and mathematicians. Meanwhile, Samuel's work on the first self-learning program, the Checkers-playing AI, demonstrated the potential of machines to improve through experience.
During the 1960s and 1970s, machine learning gained momentum as researchers developed various techniques, such as decision trees, neural networks, and genetic algorithms. These early algorithms formed the basis for more sophisticated models that we use today. However, it was the advent of powerful computers and the availability of large datasets in the late 20th century that truly propelled machine learning into the spotlight.
The Rise of Data-Driven Approaches
The turn of the millennium marked a significant shift in the machine learning landscape. With the explosion of digital data and the rise of the internet, machine learning algorithms found a fertile ground for growth. The availability of vast amounts of data, coupled with advancements in computing power, enabled researchers and practitioners to develop more complex and accurate models.
One of the key milestones in this era was the introduction of support vector machines (SVMs) in the 1990s. SVMs revolutionized pattern recognition and classification tasks, providing a powerful tool for machine learning practitioners. Additionally, the development of ensemble methods, such as random forests and gradient boosting, further enhanced the accuracy and robustness of machine learning models.
Decade | Key Developments |
---|---|
1950s | Early concepts and theories; Turing's work on AI and Samuel's Checkers AI. |
1960s-1970s | Development of decision trees, neural networks, and genetic algorithms. |
1990s | Introduction of support vector machines (SVMs) and ensemble methods. |
2000s | Explosion of digital data and the rise of deep learning. |
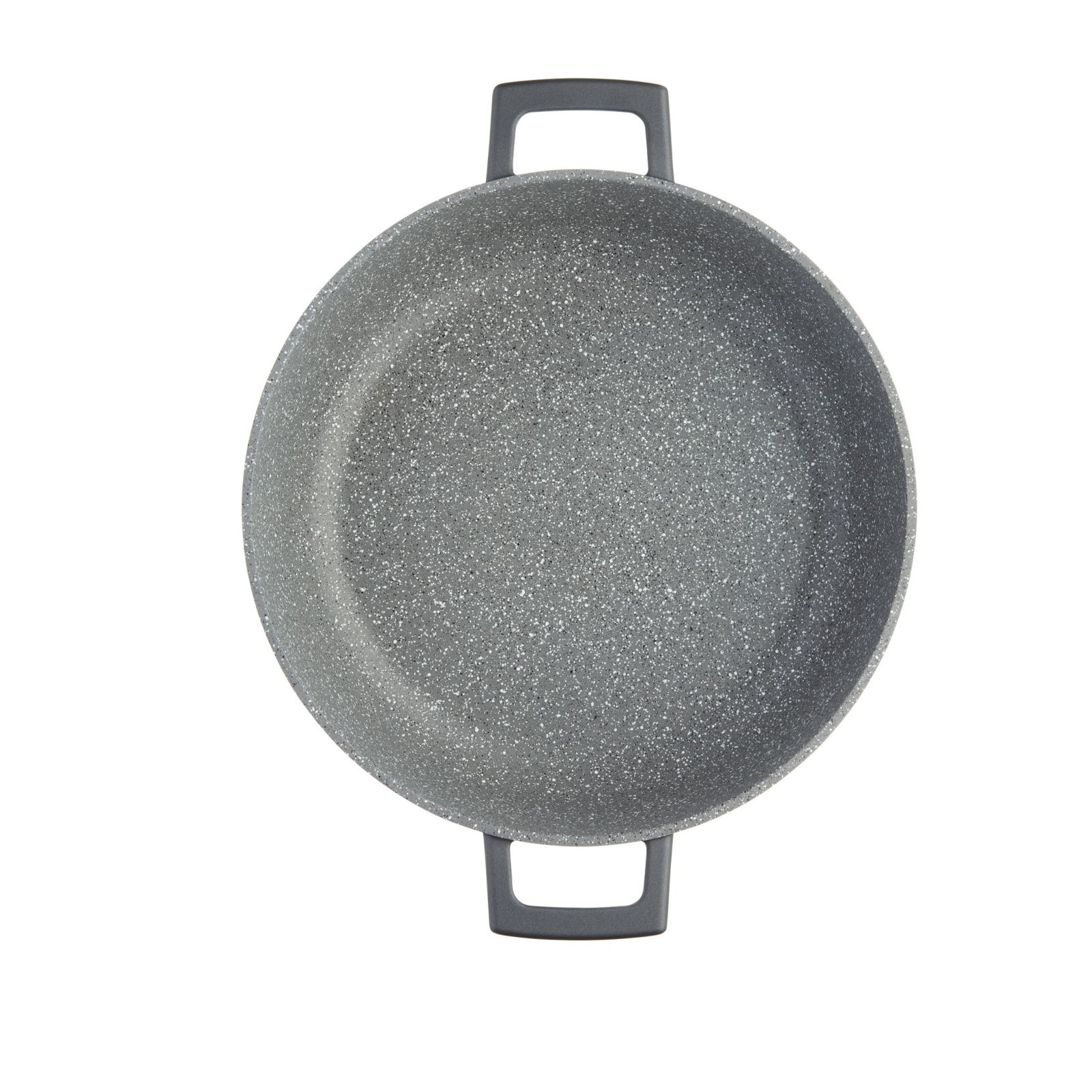
Understanding the Fundamentals of Machine Learning

At its core, machine learning is about enabling computers to learn from data and make intelligent decisions or predictions. This process involves feeding a machine learning model with labeled or unlabeled data, which it then uses to identify patterns, make connections, and develop an understanding of the underlying problem.
The Role of Data in Machine Learning
Data is the lifeblood of machine learning. It serves as the raw material that algorithms use to learn and make predictions. The quality and quantity of data significantly impact the performance and accuracy of machine learning models. Here’s a breakdown of the different types of data used in machine learning:
- Labeled Data: This type of data is already categorized or labeled, making it easier for machine learning algorithms to identify patterns and make predictions. For example, in image recognition tasks, labeled data might consist of images with corresponding labels, such as "cat" or "dog."
- Unlabeled Data: Unlabeled data is raw and uncategorized, and machine learning algorithms must identify patterns and structures within it. Unsupervised learning techniques are often employed to uncover hidden relationships and insights from unlabeled data.
- Semi-Supervised Data: Semi-supervised data is a combination of labeled and unlabeled data. It allows machine learning models to leverage the benefits of both, making it particularly useful when labeled data is scarce or expensive to obtain.
Supervised Learning: Teaching Machines with Labeled Data
Supervised learning is one of the most common approaches in machine learning. It involves training a model on a labeled dataset, where each data point has a corresponding label or output. The model learns to associate inputs with the correct outputs, enabling it to make predictions on new, unseen data.
For example, consider a supervised learning task for image classification. The model is trained on a dataset of images with corresponding labels, such as "cat," "dog," or "bird." By analyzing the labeled data, the model learns to identify patterns and features that distinguish between these classes. Once trained, it can accurately classify new images it hasn't seen before.
Unsupervised Learning: Discovering Patterns in Unlabeled Data
Unsupervised learning, on the other hand, deals with unlabeled data. In this approach, the machine learning model must identify patterns and structures on its own, without any predefined labels. Unsupervised learning is particularly useful when the goal is to uncover hidden insights or relationships within the data.
A classic example of unsupervised learning is clustering. Clustering algorithms group similar data points together based on their features, allowing us to identify natural clusters or groups within the data. This technique is widely used in customer segmentation, where businesses can identify distinct customer groups based on their behavior and preferences.
Reinforcement Learning: Learning Through Trial and Error
Reinforcement learning is a unique branch of machine learning where an agent learns to make decisions by interacting with its environment. The agent receives feedback in the form of rewards or penalties, which it uses to adjust its actions and improve its performance over time.
Reinforcement learning is particularly effective in scenarios where the goal is to optimize a specific objective, such as maximizing rewards in a game or minimizing costs in a business process. By iteratively exploring different actions and learning from the outcomes, the agent can develop a strategy that leads to the best possible outcome.
The Impact of Machine Learning Across Industries
Machine learning has permeated virtually every industry, transforming the way businesses operate and enhancing their efficiency and competitiveness. Its applications are vast and varied, ranging from healthcare and finance to transportation and entertainment.
Healthcare: Revolutionizing Patient Care and Diagnostics
In the healthcare sector, machine learning is making significant strides in improving patient care and diagnostics. Advanced algorithms can analyze medical images, such as X-rays and MRI scans, to detect anomalies and assist in the early diagnosis of diseases. Machine learning-powered systems can also predict disease outbreaks, optimize treatment plans, and personalize medicine based on individual patient characteristics.
For instance, deep learning algorithms have been used to detect early signs of breast cancer in mammograms with remarkable accuracy. By analyzing thousands of images, these algorithms can identify subtle patterns that might elude human experts, leading to earlier detection and potentially saving lives.
Finance: Enhancing Risk Assessment and Fraud Detection
The financial industry has embraced machine learning to enhance risk assessment, fraud detection, and investment strategies. Machine learning algorithms can analyze vast amounts of financial data, including market trends, customer behavior, and transaction patterns, to identify potential risks and fraudulent activities.
For example, machine learning models can detect suspicious transaction patterns, identify high-risk loans, and predict market trends with remarkable accuracy. These insights enable financial institutions to make informed decisions, mitigate risks, and improve their overall performance.
Transportation: Optimizing Logistics and Autonomous Vehicles
Machine learning plays a crucial role in optimizing transportation systems and powering autonomous vehicles. Advanced algorithms can analyze traffic patterns, optimize routing, and predict demand, leading to more efficient transportation networks.
Self-driving cars, powered by machine learning, are a prime example of this technology in action. These vehicles use a combination of sensors, cameras, and machine learning algorithms to perceive their surroundings, make decisions, and navigate safely. Machine learning enables them to recognize obstacles, understand traffic rules, and respond to dynamic road conditions, bringing us closer to a future of safe and efficient autonomous transportation.
The Future of Machine Learning: Trends and Innovations
As machine learning continues to evolve, several trends and innovations are shaping its future trajectory. Here’s a glimpse into what we can expect in the coming years:
Deep Learning and Neural Networks: Unlocking New Possibilities
Deep learning, a subset of machine learning, has gained immense popularity in recent years. Deep neural networks, inspired by the structure and function of the human brain, have demonstrated remarkable capabilities in various domains, such as image and speech recognition, natural language processing, and predictive analytics.
With the continuous advancement of deep learning algorithms and the availability of powerful computing resources, we can expect even more sophisticated applications. From improving the accuracy of medical diagnoses to enhancing the user experience of virtual assistants, deep learning will continue to unlock new possibilities and transform our daily lives.
Transfer Learning: Leveraging Pre-Trained Models
Transfer learning is an emerging trend in machine learning, where pre-trained models are fine-tuned for specific tasks. This approach leverages the knowledge and patterns learned from one domain and applies them to another, often with significantly less data and computational resources.
For instance, a pre-trained image recognition model can be fine-tuned to detect specific objects in a new dataset, reducing the need for extensive training from scratch. Transfer learning has the potential to revolutionize the development of machine learning models, especially in resource-constrained environments or when labeled data is scarce.
Explainable AI: Building Trust and Transparency
As machine learning becomes increasingly pervasive, ensuring transparency and building trust in its decisions is crucial. Explainable AI, also known as XAI, aims to provide insights into how machine learning models arrive at their decisions, making them more interpretable and trustworthy.
Explainable AI techniques, such as decision trees and rule-based models, provide a level of interpretability that helps users understand the logic behind the model's predictions. This is particularly important in sensitive domains, such as healthcare and finance, where trust and accountability are paramount.
Conclusion: Embracing the Power of Machine Learning
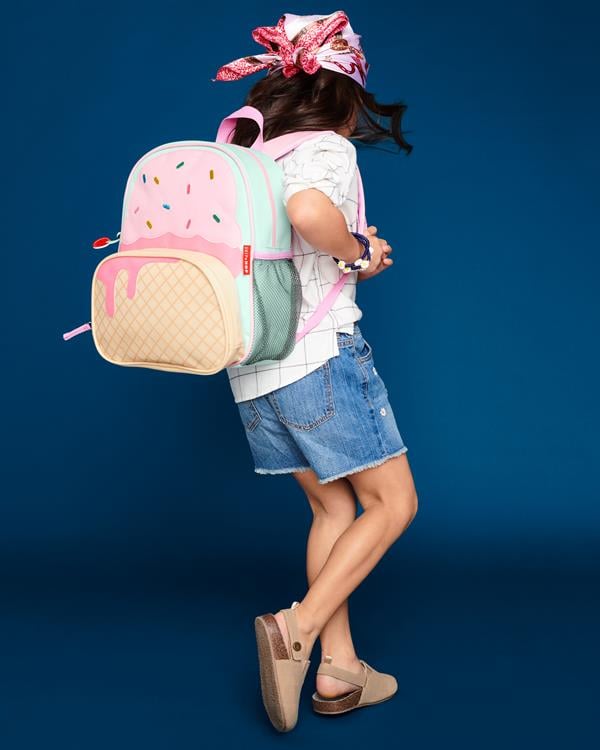
Machine learning has emerged as a transformative force, reshaping industries and revolutionizing the way we interact with technology. From its humble beginnings to its current state of rapid evolution, machine learning has proven its worth and unlocked new possibilities. As we continue to explore and innovate, the future of machine learning holds immense potential, promising a world where intelligent systems enhance our lives and drive progress.
By understanding the fundamentals of machine learning, its historical evolution, and its impact across industries, we can appreciate the profound impact this technology has had and will continue to have. As we embrace the power of machine learning, let us also strive for ethical and responsible practices, ensuring that this powerful tool is used for the betterment of society and the advancement of humanity.
How does machine learning differ from traditional programming?
+Machine learning differs from traditional programming in that it focuses on enabling computers to learn and make decisions without being explicitly programmed. Traditional programming involves writing explicit instructions for a computer to follow, whereas machine learning algorithms learn from data and make predictions or decisions based on that learning.
What are some common applications of machine learning in everyday life?
+Machine learning powers many everyday applications, such as image recognition in social media platforms, personalized recommendations in e-commerce, speech recognition in virtual assistants, and predictive analytics in smart homes. It is also used in fraud detection, medical diagnosis, and autonomous driving systems.
How can businesses leverage machine learning to gain a competitive edge?
+Businesses can leverage machine learning to gain a competitive edge by optimizing various processes and making data-driven decisions. Machine learning can enhance customer segmentation, personalize marketing campaigns, improve supply chain management, and automate repetitive tasks. Additionally, machine learning can provide valuable insights into market trends and customer behavior, enabling businesses to stay ahead of the curve.