Skip

Unveiling the Revolutionary Impact of Synthetic Data in the Age of AI

In the ever-evolving landscape of artificial intelligence (AI), synthetic data has emerged as a powerful tool, revolutionizing the way we train and develop intelligent systems. This cutting-edge technology has opened up new frontiers, offering unparalleled opportunities for innovation and progress. As we delve into the intricacies of synthetic data, we uncover its immense potential to reshape industries, enhance efficiency, and drive us toward a future defined by intelligent solutions.
Synthetic data, a term that might seem abstract to some, is an invaluable asset in the realm of AI. It refers to the creation of artificial datasets, crafted with meticulous precision to mimic real-world data. This innovative approach addresses the critical challenge of data scarcity, enabling developers to train AI models with extensive and diverse datasets, even in scenarios where acquiring real-world data is impractical, costly, or ethically challenging.
The significance of synthetic data extends far beyond mere convenience. It has become a linchpin in the quest for advanced AI solutions, particularly in scenarios where real-world data is sparse, sensitive, or simply inaccessible. By generating synthetic datasets that mirror the intricacies of the real world, developers can overcome these limitations and unlock new possibilities.
This article aims to provide an in-depth exploration of synthetic data, its applications, and its profound impact on the AI landscape. We will delve into the various techniques employed to generate synthetic data, the industries it is transforming, and the ethical considerations that accompany this powerful technology. Furthermore, we will showcase real-world examples of successful synthetic data implementation, highlighting its ability to drive innovation and improve our daily lives.
As we navigate through this comprehensive guide, we invite you to embark on a journey of discovery, unraveling the mysteries of synthetic data and its potential to shape the future of AI. Whether you are a seasoned professional in the field or a curious observer, this article promises to offer valuable insights, practical applications, and a glimpse into the exciting possibilities that synthetic data holds for the world of artificial intelligence.
Synthetic Data: Unlocking the Power of Artificial Intelligence
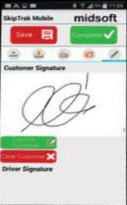
The Evolution of Synthetic Data Techniques
Synthetic data generation has come a long way since its inception, evolving from simple simulations to sophisticated techniques that can replicate complex real-world scenarios with remarkable accuracy. The journey of synthetic data techniques can be traced back to the early days of AI research, where the need for extensive datasets became apparent.
Initially, synthetic data generation involved basic simulations, often limited to simple mathematical models. These early attempts, while innovative for their time, lacked the complexity and diversity required to train robust AI models. However, they laid the foundation for future advancements and sparked the curiosity of researchers, leading to the development of more advanced techniques.
Today, synthetic data generation employs a plethora of methods, each designed to address specific challenges and requirements. Some of the key techniques include:
Generative Models: These models, such as Generative Adversarial Networks (GANs) and Variational Autoencoders (VAEs), have revolutionized synthetic data generation. They involve training a neural network to learn the underlying distribution of real data and generate new, synthetic samples that closely resemble the original data.
Rule-Based Systems: Rule-based systems utilize predefined rules and algorithms to generate synthetic data. While less flexible than generative models, they offer control and predictability, making them suitable for specific applications where data generation must adhere to strict guidelines.
Procedural Generation: This technique involves using algorithms to automatically generate synthetic data by defining rules and parameters. It is commonly used in gaming and simulation industries, where dynamic and diverse content is required.
Data Augmentation: Data augmentation techniques enhance existing datasets by applying transformations such as rotation, scaling, or adding noise. While not strictly synthetic data generation, it plays a crucial role in augmenting real-world data, making it more diverse and suitable for training AI models.
The continuous evolution of synthetic data techniques has been driven by the insatiable demand for high-quality datasets in AI research and development. As AI applications become more complex and diverse, the need for extensive, varied, and representative datasets has grown exponentially. Synthetic data generation has emerged as a key solution to this challenge, offering a flexible and scalable approach to data acquisition.
The Impact of Synthetic Data Across Industries
Synthetic data is not confined to a single domain or industry; its impact resonates across various sectors, driving innovation and efficiency. The versatility of synthetic data makes it a powerful tool for numerous applications, from autonomous vehicles to healthcare, finance, and beyond.
Autonomous Vehicles
In the realm of autonomous vehicles, synthetic data has emerged as a game-changer. Training self-driving cars requires vast amounts of data, encompassing diverse scenarios and road conditions. Acquiring such data through real-world driving can be time-consuming, expensive, and potentially dangerous. Synthetic data generation offers a solution, enabling developers to create virtual environments that mimic real-world driving conditions, allowing AI models to learn and adapt without putting human lives at risk.
Healthcare
The healthcare industry has also embraced synthetic data, particularly in medical imaging and diagnosis. Generating synthetic medical images, such as X-rays, CT scans, or MRI images, can address the scarcity of annotated medical data. This approach enables the development of advanced diagnostic tools and algorithms without compromising patient privacy or data availability. Synthetic data generation in healthcare not only accelerates research but also has the potential to improve patient outcomes and revolutionize medical practices.
Finance
The financial sector has long relied on data-driven decision-making, making synthetic data an invaluable asset. Synthetic financial data, generated to reflect real-world market conditions, enables the training of sophisticated algorithms for risk assessment, fraud detection, and investment strategies. By using synthetic data, financial institutions can test and refine their models in a controlled environment, minimizing risks associated with real-world data and ensuring regulatory compliance.
Retail and E-commerce
In the retail and e-commerce industries, synthetic data plays a crucial role in optimizing customer experiences and enhancing product recommendations. By generating synthetic customer profiles and purchase histories, retailers can train recommendation engines to offer personalized suggestions, increasing customer satisfaction and loyalty. Synthetic data generation in this context also aids in understanding customer behavior, predicting trends, and fine-tuning marketing strategies.
The impact of synthetic data extends far beyond these industries, touching upon sectors such as manufacturing, agriculture, energy, and more. Its ability to bridge the gap between data scarcity and AI advancement has made it an indispensable tool in the pursuit of intelligent solutions.
Ethical Considerations and Future Implications
While synthetic data offers immense opportunities, it also raises important ethical considerations that must be addressed. The power to generate realistic synthetic data carries the potential for misuse, particularly in areas such as deepfake generation and the manipulation of public opinion. Ensuring the responsible use of synthetic data is crucial to maintaining public trust and preventing malicious activities.
Moreover, the generation and use of synthetic data must adhere to strict privacy and data protection regulations. As synthetic data can be crafted to closely resemble real-world data, it is essential to implement robust measures to protect sensitive information and prevent unauthorized access.
Looking ahead, the future of synthetic data holds exciting possibilities. As AI continues to advance, the demand for diverse and extensive datasets will only grow. Synthetic data generation techniques are expected to become even more sophisticated, capable of generating highly realistic and nuanced datasets. This evolution will drive the development of more intelligent and accurate AI models, unlocking new frontiers in various industries.
Furthermore, the convergence of synthetic data with other cutting-edge technologies, such as edge computing and quantum computing, holds immense potential. Edge computing, for instance, can enable the generation and utilization of synthetic data in real-time, even in resource-constrained environments. Quantum computing, with its unprecedented processing power, can revolutionize synthetic data generation, enabling the creation of even more complex and realistic datasets.
Conclusion
In conclusion, synthetic data has emerged as a transformative force in the age of AI, offering unparalleled opportunities for innovation and progress. Its ability to address data scarcity, enhance efficiency, and drive intelligent solutions across industries has solidified its position as a critical enabler of AI advancement.
As we navigate the evolving landscape of synthetic data, it is crucial to approach this technology with a balance of excitement and responsibility. By embracing its potential while remaining mindful of ethical considerations, we can harness the power of synthetic data to shape a future defined by intelligent solutions, improved efficiency, and enhanced quality of life.
FAQ
How does synthetic data generation contribute to the advancement of AI?
+
Synthetic data generation plays a crucial role in the advancement of AI by providing extensive and diverse datasets for training AI models. It addresses the challenge of data scarcity, enabling developers to train robust and accurate models even in scenarios where acquiring real-world data is impractical or costly. This contributes to the development of more intelligent and efficient AI solutions across various industries.
What are some key industries benefiting from synthetic data generation?
+
Synthetic data generation has a significant impact on various industries, including autonomous vehicles, healthcare, finance, retail, and e-commerce. In autonomous vehicles, it enables the training of self-driving cars in virtual environments. In healthcare, it aids in medical imaging and diagnosis. In finance, it powers risk assessment and fraud detection algorithms. In retail and e-commerce, it optimizes customer experiences and product recommendations.
What are the ethical considerations surrounding synthetic data generation?
+
Synthetic data generation raises ethical concerns, particularly regarding its potential misuse in areas like deepfake generation and the manipulation of public opinion. It is crucial to ensure responsible use and adhere to strict privacy and data protection regulations. Synthetic data must be generated and utilized with care to maintain public trust and prevent malicious activities.
What does the future hold for synthetic data generation and AI?
+
The future of synthetic data generation and AI is promising. As AI continues to advance, the demand for diverse and extensive datasets will grow, leading to the development of more sophisticated synthetic data generation techniques. The convergence of synthetic data with technologies like edge computing and quantum computing will further revolutionize the field, enabling real-time generation and utilization of complex datasets.