Skip.

The world of technology is ever-evolving, and one of its most dynamic and fascinating aspects is the field of artificial intelligence (AI). AI has revolutionized industries, improved our daily lives, and continues to push the boundaries of what's possible. Among the myriad of AI applications, few are as intriguing and impactful as Generative AI, a subset that has captured the imagination of creators, businesses, and enthusiasts alike. This article aims to delve deep into the realm of Generative AI, exploring its origins, inner workings, applications, and future prospects.
Unveiling Generative AI: A Revolutionary Force

Generative AI is a transformative technology that empowers machines to generate new and original content, ranging from text and images to music and even entire software programs. It’s a unique branch of AI that focuses on creation rather than analysis or prediction. The power of Generative AI lies in its ability to learn from vast datasets, understand complex patterns, and then synthesize novel outputs based on that understanding.
At its core, Generative AI employs advanced machine learning techniques, particularly deep learning and neural networks, to create systems that can produce content with an impressive degree of creativity and autonomy. These systems are trained on diverse datasets, which serve as their creative palette, and then utilize this knowledge to generate new content that is often indistinguishable from human-made creations.
The journey of Generative AI began with early attempts at generating simple patterns and evolved into a sophisticated discipline, with researchers and engineers continually pushing the boundaries of what's achievable. Today, Generative AI is not just a theoretical concept but a practical tool with a wide array of applications across various sectors.
Key Components and Techniques
Several key components and techniques underpin the functionality of Generative AI models. These include:
- Generative Adversarial Networks (GANs): GANs are a type of deep neural network architecture that consists of two components: a generator and a discriminator. The generator creates new data instances, while the discriminator evaluates the authenticity of the generated data. Through an iterative process, the generator learns to create more realistic outputs, mimicking the patterns and characteristics of the training data.
- Variational Autoencoders (VAEs): VAEs are another powerful technique used in Generative AI. They learn to compress input data into a latent space and then generate new data by sampling from this space. VAEs are particularly useful for tasks like image generation and synthesis, where they can produce diverse and high-quality outputs.
- Recursive Neural Networks: These networks are designed to process sequential data, such as text or music, by recursively applying the same set of weights to each element in the sequence. They are effective for tasks like language modeling and music generation, allowing the model to capture long-range dependencies and generate coherent outputs.
Generative AI in Practice: Real-World Applications
The versatility of Generative AI is evident in its wide-ranging applications across multiple industries. Here’s a glimpse into some of the key sectors where Generative AI is making a significant impact:
Creative Industries
Generative AI has become an invaluable tool for creators and artists. In the field of visual arts, Generative AI models can generate stunning paintings, photographs, and even entire digital art collections. For example, the art generated by OpenAI’s DALL-E 2 model has garnered significant attention for its ability to create unique and visually appealing images based on text descriptions.
In music, Generative AI algorithms can compose new melodies, harmonies, and even entire musical pieces. These models can learn from existing musical styles, genres, and compositions to create original music that aligns with specific artistic directions. Additionally, Generative AI is being used to generate special effects, animations, and visual effects for movies and video games, enhancing the immersive experience for audiences.
Creative Industry | AI Application |
---|---|
Visual Arts | Generative Art, Image Generation |
Music | Music Composition, Sound Synthesis |
Special Effects | Visual Effects Generation, Animation |
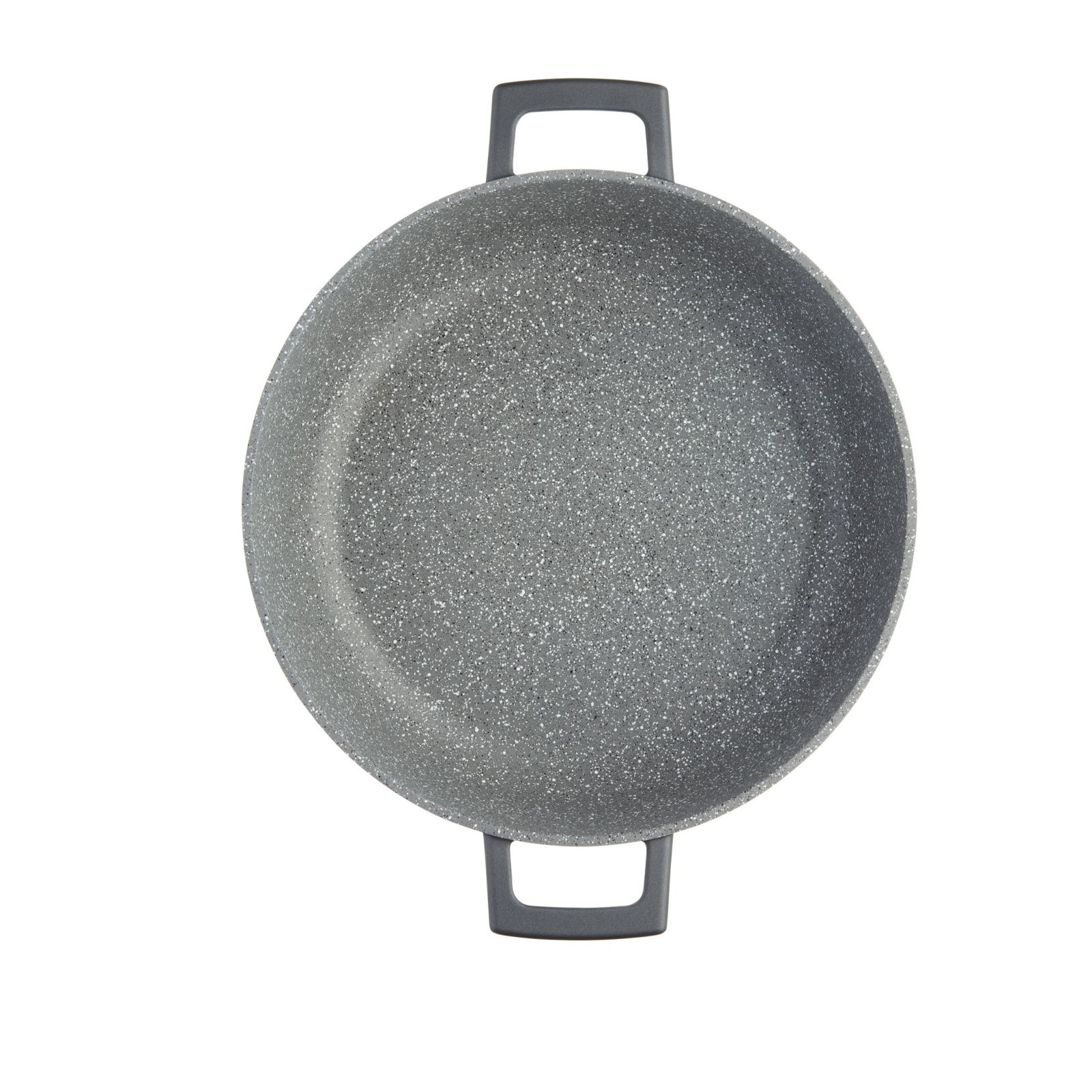
Healthcare and Medical Research
The healthcare sector is harnessing the power of Generative AI to accelerate drug discovery and development. Generative AI models can predict and design new molecular structures with potential therapeutic properties, aiding in the identification of novel drug candidates. These models can analyze vast chemical databases and generate molecules that meet specific criteria, significantly speeding up the drug discovery process.
Furthermore, Generative AI is being used in medical imaging for tasks like image segmentation and synthesis. These applications can assist in diagnosing diseases, planning surgical procedures, and even training medical professionals through the generation of realistic medical scenarios.
Healthcare Application | AI Impact |
---|---|
Drug Discovery | Molecular Design, Therapeutic Candidate Identification |
Medical Imaging | Image Segmentation, Synthesis for Diagnosis and Training |
Manufacturing and Design
Generative AI is transforming the manufacturing and design industries by optimizing processes and enhancing creativity. In product design, Generative AI can generate a vast array of design options based on specific criteria, such as material properties, manufacturing constraints, or aesthetic preferences. This not only speeds up the design process but also allows for the exploration of a broader design space, leading to innovative and optimized solutions.
Additionally, Generative AI is being used in process optimization, where it can identify bottlenecks and inefficiencies in manufacturing processes. By simulating and analyzing various scenarios, Generative AI models can suggest improvements, reduce costs, and increase productivity.
Entertainment and Media
The entertainment industry is embracing Generative AI to create engaging and immersive experiences. In video games, Generative AI can generate dynamic and diverse game content, such as procedurally generated landscapes, character designs, and story elements. This not only reduces the need for manual content creation but also adds a layer of unpredictability and replayability to games.
In the film industry, Generative AI is being used to create realistic digital characters, environments, and special effects, reducing the time and resources required for traditional animation and visual effects techniques. Additionally, Generative AI is enabling the personalization of media content, such as generating personalized movie recommendations or custom video game experiences tailored to individual preferences.
Finance and Economics
Generative AI is playing a pivotal role in financial markets and economic forecasting. These models can analyze vast amounts of financial data, market trends, and economic indicators to generate predictions and scenarios. They are used for tasks like portfolio optimization, risk assessment, and market strategy development, helping financial institutions make more informed decisions.
Furthermore, Generative AI is being applied to synthetic data generation, which is crucial for training and testing financial models. By creating realistic yet artificial financial data, Generative AI ensures that models are robust and can handle a wide range of market conditions.
Performance and Limitations of Generative AI

While Generative AI has achieved remarkable feats, it’s essential to understand its performance characteristics and limitations. One of the key strengths of Generative AI is its ability to produce diverse and high-quality outputs. Models like GANs and VAEs can generate content that is often indistinguishable from human-made creations, thanks to their ability to learn and mimic complex patterns from training data.
However, Generative AI also faces certain challenges. One of the primary concerns is the need for large and diverse training datasets. Insufficient or biased data can lead to biased or limited outputs, impacting the quality and diversity of the generated content. Additionally, the interpretability of Generative AI models can be a challenge, as it's often difficult to understand why a model generates a particular output, making it hard to diagnose and rectify errors.
Another consideration is the computational intensity of Generative AI models. Training and running these models can require significant computational resources, which may limit their accessibility and scalability, especially for smaller organizations or individuals.
Ethical and Societal Considerations
As with any powerful technology, Generative AI raises important ethical and societal questions. One of the primary concerns is the potential for misuse, such as generating deepfake content for malicious purposes. The ability of Generative AI to create highly realistic and convincing outputs can be exploited for spreading misinformation, identity theft, or other harmful activities.
Another ethical consideration is the impact of Generative AI on creative industries and the potential displacement of human workers. While Generative AI can enhance creativity and productivity, there are concerns about its potential to replace human artists, writers, and designers. Balancing the benefits of Generative AI with the need to protect human creativity and livelihoods is a complex challenge that requires careful consideration and regulation.
Additionally, Generative AI raises questions about intellectual property and copyright. As Generative AI models can create entirely new and original content, it's important to establish clear guidelines and frameworks for determining ownership and attribution of generated works.
Future Prospects and Developments
The future of Generative AI is promising, with ongoing research and development pushing the boundaries of what’s possible. Several key trends and developments are shaping the future of this technology.
Advancements in Model Architecture
Researchers are continually developing more sophisticated and efficient model architectures for Generative AI. These advancements aim to improve the quality and diversity of generated outputs while reducing the computational resources required. Some of the key areas of focus include:
- Improved Training Techniques: Researchers are exploring more effective training methods, such as transfer learning and meta-learning, to enhance the performance of Generative AI models.
- Attention Mechanisms: Incorporating attention mechanisms into Generative AI models can help them focus on relevant parts of the input data, improving the quality and coherence of generated outputs.
- Multi-Modal Learning: By training models on multiple data modalities, such as text, images, and audio, Generative AI can become more versatile and capable of generating outputs across different media types.
Integration with Other AI Disciplines
Generative AI is increasingly being integrated with other AI disciplines to create more powerful and holistic systems. For example, combining Generative AI with reinforcement learning can enable the creation of agents that can learn and generate content in dynamic and interactive environments. This integration has applications in areas like robotics, where agents can learn to navigate and interact with their surroundings in real-time.
Exploring New Applications
The potential applications of Generative AI are vast and continue to be explored. Some emerging areas of interest include:
- Generative AI for Natural Language Processing (NLP): Generative AI is being applied to NLP tasks, such as text generation and summarization, to create more coherent and contextually relevant outputs. This has implications for content creation, customer service chatbots, and language translation.
- Healthcare Diagnostics: Generative AI is being explored for its potential to assist in medical diagnostics. By generating synthetic medical images and scenarios, Generative AI can help train and improve the accuracy of diagnostic models, particularly in rare or complex cases.
- Creative Collaboration: Generative AI is being used to collaborate with human creators, providing inspiration and suggestions to enhance their work. This collaborative approach has the potential to unlock new creative possibilities and streamline the creative process.
Addressing Ethical and Societal Concerns
As Generative AI advances, addressing ethical and societal concerns becomes increasingly important. Researchers, developers, and policymakers are working together to establish guidelines and frameworks for the responsible use of Generative AI. This includes developing techniques to detect and mitigate deepfakes, ensuring data privacy and security, and establishing regulations for intellectual property and copyright.
Democratizing Access to Generative AI
One of the key challenges in the widespread adoption of Generative AI is its computational intensity and resource requirements. However, ongoing efforts are focused on making Generative AI more accessible and user-friendly. This includes the development of lightweight models that can run on less powerful hardware, as well as the creation of user-friendly interfaces and tools that enable non-technical users to harness the power of Generative AI.
Conclusion
Generative AI is a powerful and transformative technology with the potential to revolutionize a wide range of industries and applications. From enhancing creativity and productivity to accelerating drug discovery and optimizing manufacturing processes, Generative AI is a force that is shaping the future. While it faces challenges and ethical considerations, the ongoing advancements and responsible development of Generative AI hold the promise of a brighter and more innovative future.
As we continue to explore and harness the potential of Generative AI, it's essential to remain mindful of its capabilities and limitations. By understanding and addressing these aspects, we can ensure that Generative AI is used for the betterment of society, unlocking new possibilities and driving progress in ways we've only begun to imagine.
What is Generative AI, and how does it differ from other types of AI?
+Generative AI is a subset of Artificial Intelligence that focuses on creating new and original content, such as text, images, music, and even software programs. Unlike other types of AI that analyze or predict data, Generative AI aims to generate novel outputs based on learned patterns from training data.
What are some key applications of Generative AI in the real world?
+Generative AI has a wide range of applications across industries. In creative fields, it’s used for generating art, music, and special effects. In healthcare, it aids drug discovery and medical imaging. In manufacturing, it optimizes processes and designs. In entertainment, it creates dynamic game content and realistic digital characters. And in finance, it’s used for market predictions and synthetic data generation.
What are the key challenges and limitations of Generative AI?
+Generative AI faces challenges such as the need for large and diverse training datasets, interpretability issues, and computational intensity. Additionally, there are ethical concerns regarding misuse, the potential displacement of human workers, and intellectual property rights.
What are some future trends and developments in Generative AI?
+Future trends in Generative AI include advancements in model architecture, such as improved training techniques and multi-modal learning. Integration with other AI disciplines, like reinforcement learning, is also gaining traction. Additionally, new applications are being explored, such as Generative AI for NLP and healthcare diagnostics. Ethical considerations and the democratization of access to Generative AI are also key areas of focus.